Research Seminar in Economics, May 14
.
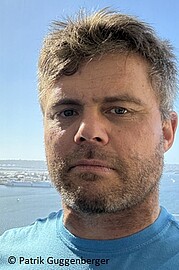
Given the lack of analytical solutions for minimax regret treatment rules in most scenarios of empirical interest, finding numerical approximations is of key interest. To do so when potential outcomes are in {0, 1} we suggest discretizing the action space of nature and applying an algorithm based on Robinson’s (1951) pioneering work on iterative solutions for two-person zero sum games with finite action space. Crucially, an application of Bayes’ rule avoids the need to evaluate regret of each candidate treatment rule in each iteration. After every iteration of the algorithm we obtain a bound for the distance of the maximal regret of the current treatment rule to the minimax regret value. In the case where potential outcomes are in [0, 1] we apply the coarsening approach to the approximation of a minimax regret rule from the binary case. As one application we consider a policymaker who has to choose between two treatments after observing a dataset with potentially unequal sample sizes per treatment. To significantly decrease computation time we leverage the general algorithm with theoretical insights about certain symmetry conditions that can be imposed on the treatment rules. As another application, we consider testing several innovations against the status quo.